AI-generated user testing
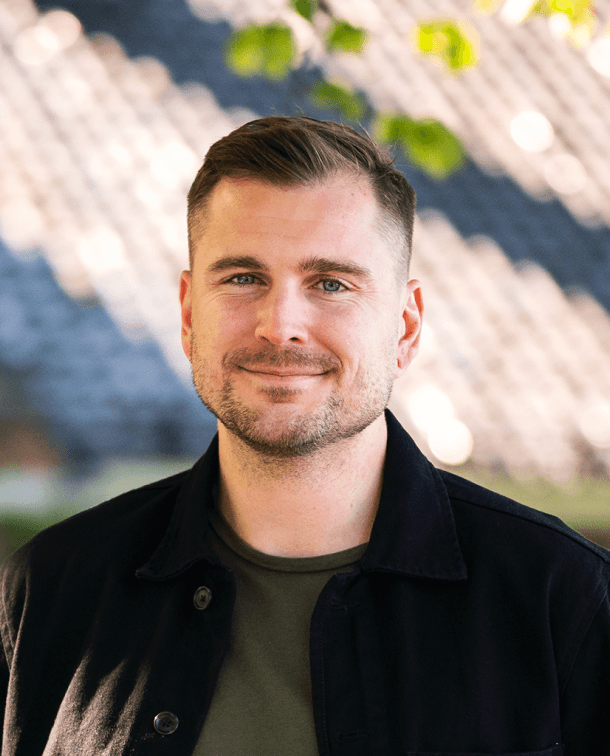
Published:
Recently, I discovered an AI-based eye tracker plugin for Figma, which allows you to test designs to predict where users attention is likely to go. The accuracy is up to 96%, and you don't need physical participants for testing. Sounds quite impressive, doesn't it?
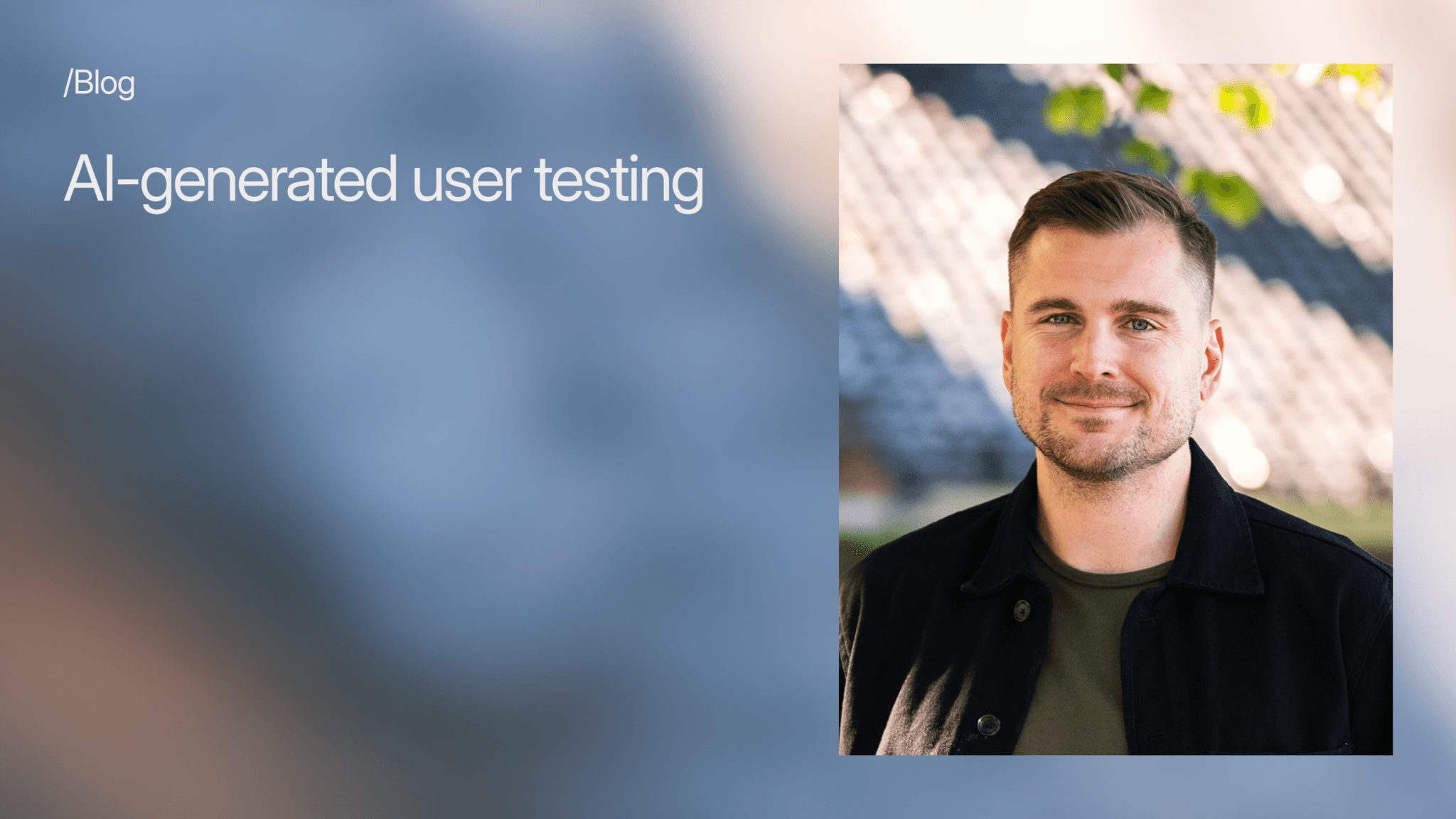
10 critical seconds
You might be wondering why you should use a tool like Attention Insight – don't you already have enough plugins?
The answer is simple: Because your users won't stay very long on your website.
Many visitors leave a webpage after 10-20 seconds, but with a clear value proposition, the likelihood that you keep them longer increases. To capture attention, you must communicate this clearly and succinctly within the first few seconds. Your design must be optimized for attention to achieve this.
Increase design usability with AI
When we read, we scan superficially and read only parts of the content, in fact, no more than 20-28% of the text. It goes without saying that it becomes important to optimize content for this reading pattern.
- Attention Insight helps you identify design errors earlier, allowing you to achieve better-performing designs from the start. This is done using four features in the plugin module:
- Generate a thermographic map showing attention/focus.
- AOF (Areas of Interest), giving you the percentage of attention for a defined area of interest.
- Compare designs for different versions (A/B testing).
- Score on the design's clarity.
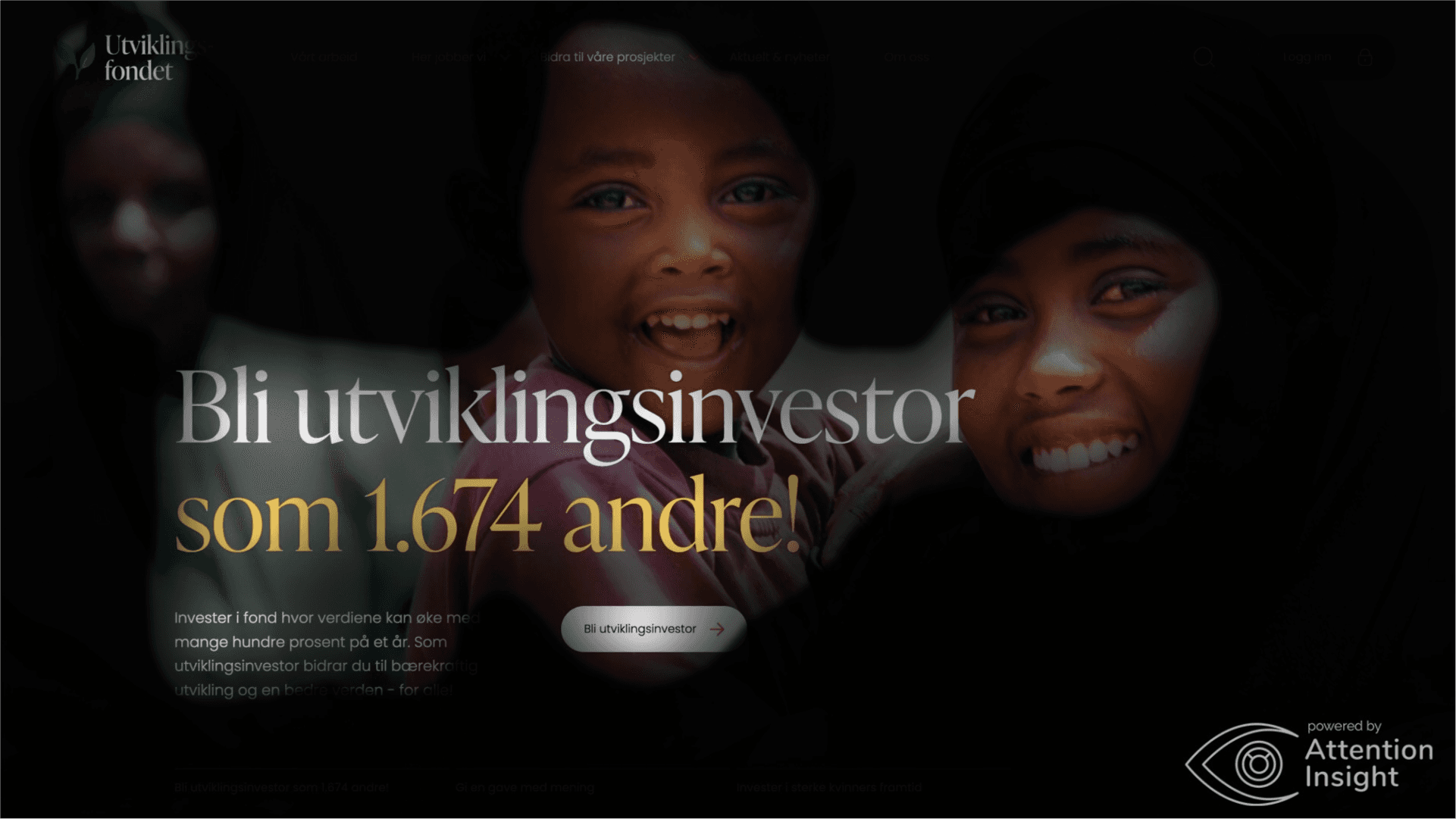
Focus map to see where your users focus is more likely to be
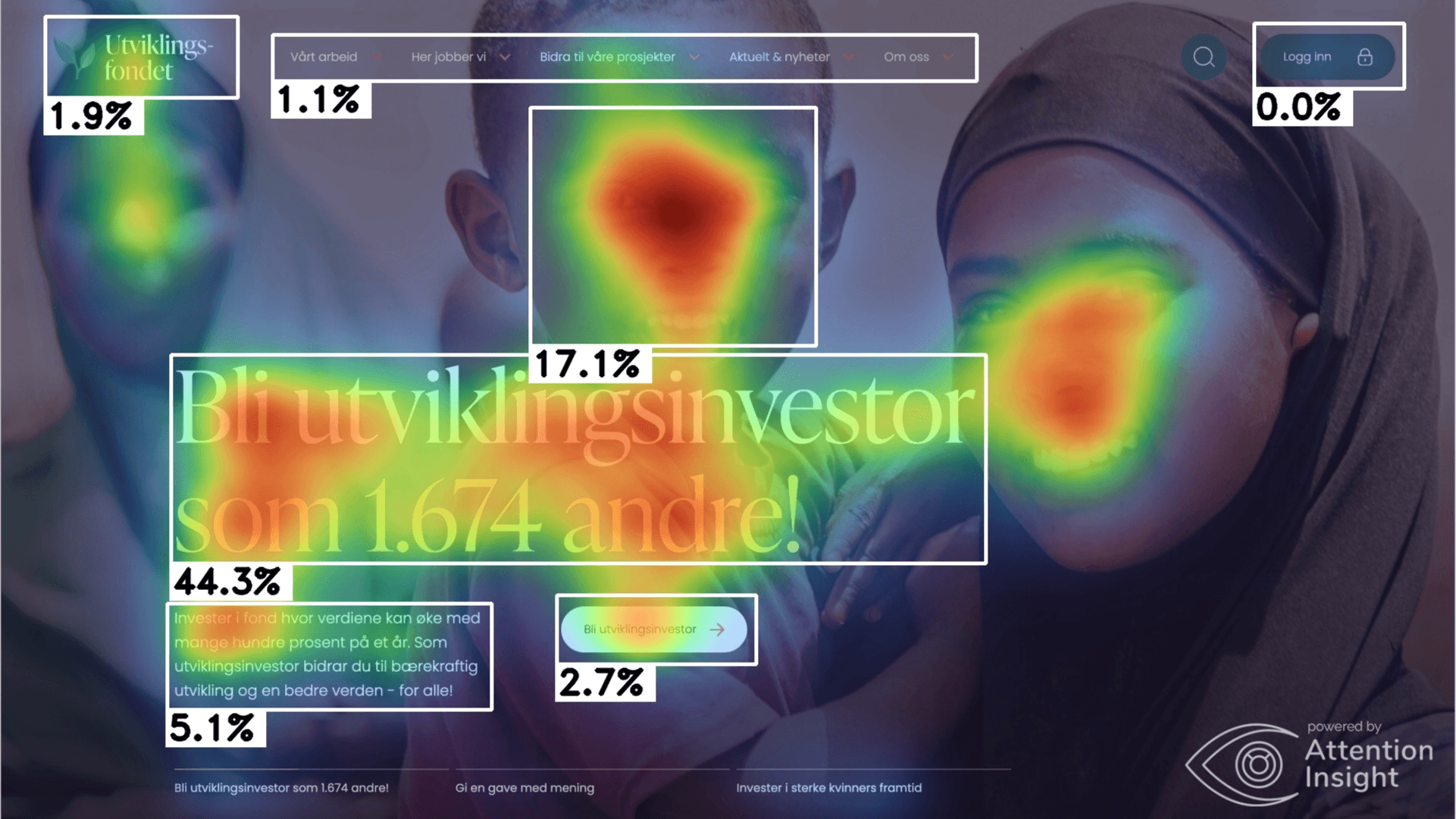
A heatmap showing areas of interest (AOF)
A quick overview of the technology
An overview of the technology behind Attention Insight can make it easier to understand how it can make our designs more effective.
Eye tracking: The method where one maps the eye's movement pattern. Here, Attention Insight collaborated with a neuromarketing laboratory that is a member of the International Neuromarketing Science and Business Association (NMSBA).
Dataset: The algorithm is trained on eye tracking datasets from over 70,000 people. The duration of attention is four seconds, the gender distribution is 58% women and 42% men, the average age is in the 21-30 year segment, and the majority of participants are from the USA and Europe.
Algorithm: The result we get is created by a deep learning algorithm called Convolutional Neural Network (CNN). CNN is a computational system with an architecture that simulates the biological brain and mimics how our neuronal layers work.
Accuracy: To assess how accurate Attention Insight is, the results of 300 images were sent to the Massachusetts Institute of Technology (MIT)/Tuebingen saliency benchmark. The results showed an impressive accuracy of 90-96% for thermographic maps across all designs.
Not your real users, but a good indication
Using tools like this, especially in projects where user testing is not included in the budget, can help you launch better-performing designs from the start. And in the future, I believe we will see more AI-powered tools helping us make design decisions.
But remember...
You are not testing with real people, so the use of this type of tool should serve as an indicator and not a source of truth. And finally, to get the best out of your results, it is important to plan and structure your tests well.